Momentum for digital technologies in lab research is growing and was accelerated by the COVID-19 pandemic, which caused teams to rapidly adopt digital tools and rethink their current processes. While there are many digital use cases and technologies available to enhance lab research, they can be broadly broken up into three categories: modeling and informatics, knowledge management, and lab automation.
Based on primary interviews, we identified key technologies and use cases for each segment that range from those that are ready to adopt now (e.g., property optimization) to those that should be explored or monitored (e.g., quantum computing). Overall, we found modeling and informatics to have the greatest impact and expect lab automation to take longer to mature. Lastly, we found that cultural barriers pose as significant a challenge to adoption as technology barriers do.
LAB EFFICIENCY DECLINES, WHILE INNOVATION CHALLENGES EMERGE
The digital transformation of innovation and R&D is a theme that Lux has covered before. While innovation and R&D consists of many activities, this article specifically dives deeper into lab research and takes a fresh look at where the key digital developments are occurring. These digital solutions are sorely needed; they come at a time when industries that make heavy use of lab research, such as chemicals and pharmaceuticals, continue to face declining productivity on top of the new challenges.
- Rising costs: The rising expense of materials, instruments, personnel, and regulatory compliance, coupled with flat or decreased R&D spending, means that companies increasingly need to find ways to do more with less.
- Environmental factors: Industrywide pushes toward developing more sustainable products and environmentally friendly production processes add costs and constraints to the R&D process.
- Long development cycles: An average material development cycle can take between ten and twenty years. With customers demanding customized products, these long development cycles are becoming unviable.
- Information overload:The volume of research data generated continues to grow exponentially every year. Organizations, however, lack adequate methods for systematically managing and deriving value from this data.
MOMENTUM FOR THE DIGITAL LAB IS GROWING
Visions for the lab of the future have evolved over the past few decades, and while individual instruments and processes have benefited from digital tools like automation and analytics, it’s safe to say that lab research has changed far less than many would have predicted. However, with an increasingly broad and powerful digital toolkit that includes tools like AI, robotics, and IoT sensors, the lab of the future—one that is significantly more automated, efficient, and effective—may be closer to reality.
After an initial wave of hype and activity in the late 1990s, innovation interest in applying digital tools to the lab plateaued for nearly a decade. However, starting around 2013, there has been a steady growth in innovation interest, showing that the space may be in a phase where it could lead to a significant impact.
THREE CORE CATEGORIES OF EMERGING DIGITAL LAB TOOLS
While there are many digital use cases and technologies available to enhance lab research, they fall into three broad categories: modeling and informatics, knowledge management, and lab automation. While each category has its own defining features, there is overlap and synergy between the categories. For example, lab automation can be used to collect experimental data, which can then be fed into informatics systems to ultimately generate knowledge. Below and on the following slides, we describe these categories in more detail.
Modeling and Informatics
Using modeling and informatics tools like machine learning to accelerate the development and discovery process. Example: Using machine learning to model and predict polymer properties in order to shorten the overall polymer design time.
- Optimization: Optimizing in silico or designing experiments to optimize existing chemical and/or material structures for a specific structure, properties, performance, etc. Informatics vendors typically focus on optimization, given the strong commercial interest and technical feasibility.
- Discovery: Discovering wholly new chemicals and materials, including synthetic routes. While this is in earlier stages in the materials space, biotech and pharmaceutical companies are very active here.
Knowledge Management
Systematically capturing, analyzing, and distributing knowledge throughout an R&D organization. Example: Using natural language processing (NLP) to sift through published literature in order to identify efficient reaction pathways.
- Documentation and collaboration: Recording notes and data and enabling more seamless collaboration. Vendors in this space tend to focus on electronic notebooks and laboratory information management systems (LIMS).
- Knowledge search: Searching, analyzing, and extracting insights from internal and external knowledge like research papers and reports. While some companies like Sinequa offer more generic search capabilities, others like Patsnapfocus specifically on patent data.
Lab Automation
Automating physical experimentation through robotics as well as data collection and lab management through IoT and connected sensors. Example: Using robotics to enable high-throughput testing and screening of materials.
- Conducting experiments: Automating experimentation steps. Players in this space include those selling hardware or orchestration software as well as those using automation for their own research.
- Lab management and data extraction: Monitoring and maintaining lab instruments and conditions as well as automating the extraction and integration of data from instruments. Vendors in this space include those that sell connected IoT devices as well as software players focused on data extraction.
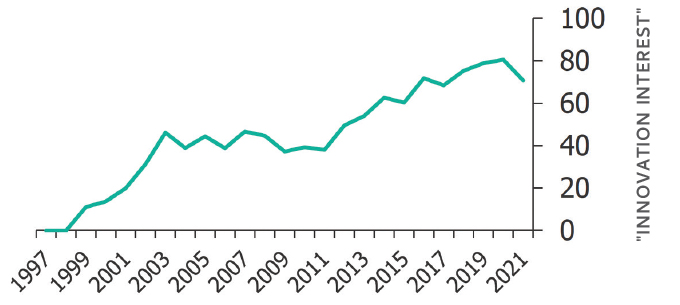
OPTIMIZATION USE CASES
Modeling and informatics approaches tend to have the greatest impact in optimization use cases, where existing chemical and material structures are optimized or screened using techniques like machine learning. In particular, the optimization of small molecules, polymer-based formulations, and multiphase alloy development has some of the highest levels of activity. In many cases, companies are using these approaches to optimize the design of experiments to enable a feedback loop between traditional bench experiments and digital.
Discovering and designing new chemicals and materials from scratch—for example, by using generative machine learning models—will be the next major outcome for modeling and informatics. Already, this is starting to occur in the small molecule space, and it will begin to impact larger and more complex molecules in the coming years. Additionally, while quantum chemistry is often simulated using conventional computers, quantum computing (QC) holds significant potential to enable more accurate simulations of large molecules. The timeline for the impact of QC will depend largely on hardware developments; however, many companies are already active in this space.
CONNECTING INFORMATION AND CAPTURING TACIT KNOWLEDGE
Over the past decade, there’s been a significant rise in the use of electronic lab notebooks (ELNs) as well laboratory information management systems (LIMS) to track notes, experiments, samples, and more. More recently, companies have been adopting tools that enable researchers to search over past reports and find employees with the right expertise. Overall, companies tend to see moderate impact from the use of digital knowledge management tools.
There are three major areas of future opportunity for knowledge management. The first is digitizing sources of information that still remain analog. For example, all meetings could soon be recorded, transcribed, and analyzed for insights. The second is in connecting information sources, not just from R&D but from other business areas like manufacturing. AI tools can then automatically extract data, identify novel connections between data points, and provide intuitive interfaces for researchers to easily find what they’re looking for. Finally, companies are increasingly looking to invest in tools and processes that enable the capture of tacit knowledge, although this area is still in its early stages and will require experimentation.
NEW APPROACHES TO LAB AUTOMATION
There are two major areas of impact within lab automation. The first is automating simple, repetitive physical tasks with tools and processes like high-throughput screening for testing large numbers of compounds. The second is automatically extracting data from lab equipment and instruments. Historically, pulling this data from equipment required customized scripts and integrations, but companies are actively looking to automate this process.
On the physical automation side, researchers are increasingly looking to adopt automated synthesis and flow chemistry systems for automating more complex tasks. While these efforts are still in early stages, now is the right time to begin experimenting with such solutions. Additionally, building on high-throughput screening techniques, some companies are looking toward “closed-loop” automation, where experimentation is done and decisions are made in an automated feedback loop. While promising, clients should monitor activity until it is validated further. Likewise, some researchers are utilizing autonomous mobile lab robots; however, their benefit is still questionable. Finally, companies are starting to adopt IoT sensors for automated lab management and environmental parameter monitoring.
THE COMBINED ROADMAP TO THE LAB OF THE FUTURE
If you refer back to figure 1, we show key digital technologies and use cases mapped onto the radar roadmap. Across the three segments, there are technologies that clients should be adopting today, such as material informatics for property optimization, electronic lab notebooks, and automated data extraction tools. We also show much earlier-stage technologies that clients should be actively monitoring or exploring, such as quantum computing, voice AI, and closed-loop automation, which will play an important role five to ten years from now, as well as technologies in-between these two extremes. Overall, clients should customize this roadmap to plot a course for their lab of the future.
While each segment of digital in the lab has unique value propositions, modeling and informatics will likely provide the greatest near-term impact in terms of ROI and technical viability. Within this space, clients should focus not on trying to eliminate physical experimentation or conducting more experiments faster but rather on conducting the right experiments using various optimization techniques. Looking toward the future, two significant opportunities are in de novo molecule design and in quantum computing for improving molecular simulations. Companies that are currently bottlenecked by conventional computers should explore early partnerships with quantum computing organizations, while others should closely monitor the technology to identify the right point to jump in.
Knowledge management and lab automation will also play a critical role in the lab of the future. In knowledge management, clients should focus on breaking down data silos and using technologies like NLP to make knowledge creation and accessibility frictionless throughout an organization. While some areas of lab automation, such as high-throughput screening, are becoming more mature, clients should expect complex capabilities like automated synthesis to disappoint in the near term, as the technology still isn’t mature or scalable.
Finally, as mentioned throughout this report, culture and process changes are as critical as the technologies for creating a significant impact. While it’s a difficult problem, clients should first focus on identifying the right KPIs and metrics that can guide their digital efforts to success (these can include performance improvements, researcher hours saved, time to market, etc.). Ultimately, while many traditional scientists may be skeptical of digital tools, true digital transformation occurs when there is both buy-in at the top and a bottom-up push from employees to adopt these tools as part of their workflows. Start small to gain traction and gather results. The best way to convince skeptical scientists and researchers of the value of these tools is to come armed with data. Clients should also work directly with the researchers and technicians in the lab to design these digital tools to further increase buy-in and adoption.
DIGITAL WILL BECOME A KEY COMPETITIVE ADVANTAGE
Currently, the three segments of modeling and informatics, knowledge management, and lab automation often operate in somewhat separate ecosystems; however, we expect the lines between these areas to grow increasingly blurry over time. As part of this process, we expect to see consolidation in this space, where materials informatics companies may acquire a lab notebook or lab automation company. While clients should seek to avoid lock-in to specific vendors, vendors that create ecosystems of tools that interoperate and share data seamlessly will be well-positioned in this space.
As R&D becomes increasingly digitized, we also expect to see more overlap among research, product development, manufacturing, sales (e.g., with digital sales platforms), etc. Instead of just predicting the property of a specific formulation, companies will try to predict the eventual cost to produce the product, where and how it could be manufactured, how long it will take to produce, etc. Once these steps are all digitized, companies can optimize each part of the process and cut down the time it takes to go from research to finished products from years to months or even weeks.
While there is no shortage of near-term challenges in this space, we expect digital capabilities to provide a significant competitive advantage longer-term. These data-driven capabilities will compound over time as companies collect more data and build better relationships with customers by understanding their needs through data. Eventually, those who effectively invest in and adopt these tools will be able to operate at a speed and level of effectiveness that will be unmatched by those who do not. While clients should start by working with vendors to adopt digital tools, they should also have an eye toward bringing these capabilities in-house to build up a core competency in this space.
By Cole McCollum, Shriram Ramanathan, and Kevin See, Lux Research
FOR MORE INFORMATION
Siemens Digital Industries Software, a business unit of Siemens Digital Industries, is a leading global provider of software solutions to drive the digital transformation of industry, creating new opportunities for manufacturers to realize innovation. With headquarters in Plano, Texas, and over 140,000 customers worldwide, we work with companies of all sizes to transform the way ideas come to life, the way products are realized, and the way products and assets in operation are used and understood. For more information, visit www.sw.siemens.com.
MODERN PUMPING TODAY, January 2022
Did you enjoy this article?
Subscribe to the FREE Digital Edition of Modern Pumping Today Magazine!
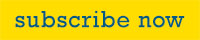